Can an algorithm identify disease-carrying genetic mutations to help predict survival?
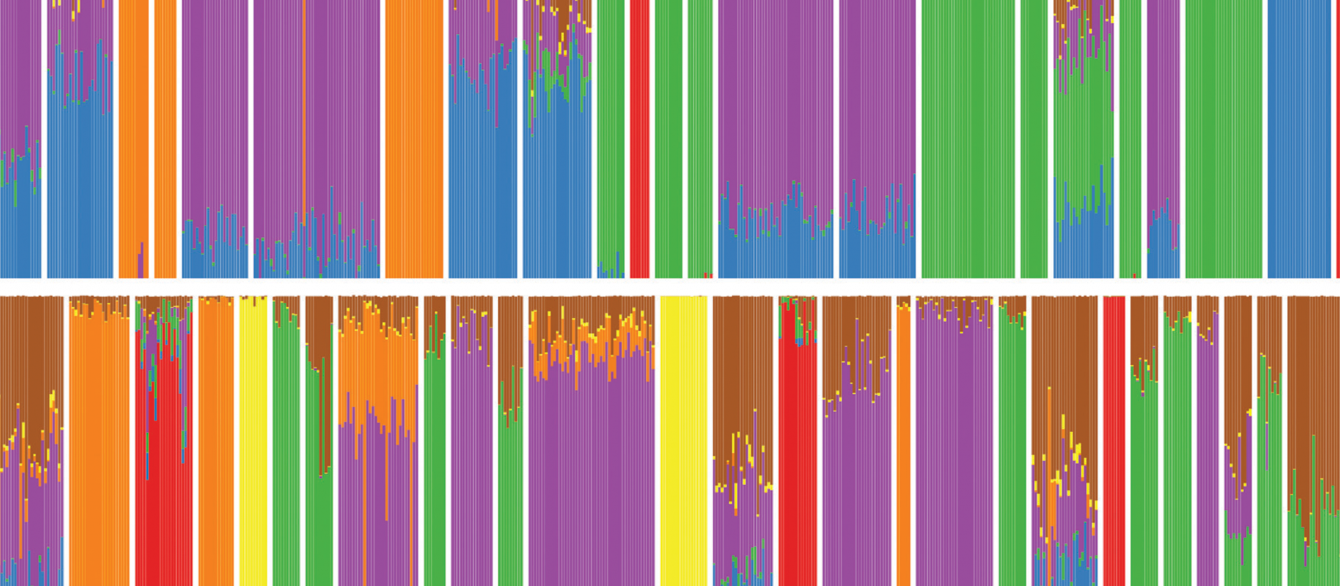
Genetic data can help predict an individual’s likelihood of getting certain diseases. These predictions would rapidly advance treatment and prevention. However, the staggering amount of data from ancestral populations around the world can be difficult to analyze.
A team of researchers led by Columbia computer science professor David Blei has developed a new machine-learning algorithm that can scan enormous quantities of genetic data randomly dispersed across populations.
On simulated data sets of 10,000 people, the algorithm, dubbed TeraStructure, could estimate population structure twice as fast as current state-of-the-art algorithms. TeraStructure could analyze the genomes of one million individuals, well beyond modern software capabilities, and potentially characterize the structure of world-scale human populations.
Through analyzing massive genetic data sets, these new algorithms can help identify disease-carrying mutations; by analyzing health records, they can help predict an individual’s survival.
Make Your Commitment Today